2023 article
Compositional Mixed-Criticality Systems with Multiple Executions and Resource-Budgets Model
2023 IEEE 29TH REAL-TIME AND EMBEDDED TECHNOLOGY AND APPLICATIONS SYMPOSIUM, RTAS, pp. 67–79.
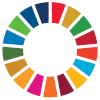
Software reusability and system modularity are key features of modern autonomous systems. As a consequence, there is a rapid shift towards hierarchical and compositional architecture, as evidenced by AUTOSAR in automobiles and ROS2 in robotics. The resource-budget supply model is widely applied in the real-time analysis of such systems. Meanwhile, real-time systems with multiple critical levels have received significant attention from the research community and industry. These systems are designed with multiple execution budgets for multiple system-critical levels. Existing studies on mixedcriticality systems consider a dedicated resource supply. This paper considers a novel generalized system model with multiple execution estimations and resource-budget supplies for compositional systems. An analytical model and a demand-bound function-based schedulability test are presented for the EDFbased scheduler in the proposed compositional mixed-criticality system. A range for setting the resource supply period is derived to ensure the schedulability of workloads when supply budgets are known. The general performance of the scheduling framework and its wider applicability is further demonstrated and evaluated using synthetic workloads and resource models, where synthetic workload parameters are derived through a case study on an autonomous driving system.