2023 article
Upscaling soil organic carbon measurements at the continental scale using multivariate clustering analysis and machine learning
Wang, Z., Kumar, J., Weintraub-Leff, S. R., Todd-Brown, K., Mishra, U., & Sihi, D. (2023, July 8).
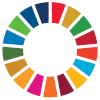
Estimates of soil organic carbon (SOC) stocks are essential for many environmental applications. However, significant inconsistencies exist in SOC stock estimates for the U.S. across current SOC maps. We propose an upscaling framework that combines unsupervised multivariate geographic clustering (MGC) and supervised random forest regression, improving SOC maps by capturing heterogeneous relationships with SOC drivers. We first used MGC to divide the U.S. into 20 SOC regions based on the similarity of covariates (soil biogeochemical, bioclimatic, biological, and physiographic variables). Subsequently, separate random forest models were trained for each SOC region, utilizing environmental covariates and SOC observations. Our estimated SOC stocks for the U.S. (52.6 + 3.2 Pg for 0-30 cm and 108.3 + 8.2 Pg 0-100 cm depths) were within the range estimated by existing products like HWSD (46.7 Pg for 0-30 cm and 90.7 Pg 0-100 cm depth) and SoilGrids 2.0 (45.7 Pg for 0-30 cm and 133.0 Pg 0-100 cm depth). However, independent validation with soil profile data from the National Ecological Observatory Network showed that our approach (R2 = 0.51) outperformed the estimates obtained from Harmonized World Soil Database (R2 = 0.23) and SoilGrids 2.0 (R2 = 0.39) for the topsoil (0-30 cm). Uncertainty analysis (e.g., low representativeness and high coefficients of variation) identified regions requiring more measurements, such as Alaska and the deserts of the U.S. Southwest. Our approach effectively captures the heterogenous relationships between widely available predictors and SOC across regions, offering reliable gridded SOC estimates for benchmarking Earth system models.