2019 journal article
Estimation of evapotranspiration and its parameters for pine, switchgrass, and intercropping with remotely-sensed images based geospatial modeling
ENVIRONMENTAL MODELLING & SOFTWARE, 121.
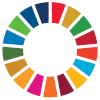
Intercropping switchgrass (Panicum virgatum) with pine can increase bioenergy feedstock production without land opportunity costs but can potentially alter water budgets. Measuring evapotranspiration (ET) and its parameters (stomatal conductance (gs), leaf area index (LAI), canopy temperature (Tc), and soil moisture (SM)) across cropping systems is costly and time-consuming. However, interpretation of remotely-sensed data can facilitate the effective assessment of relative ET demands among competing forest landuses. This study develops and tests geospatial models informed by a normalized difference vegetation index (NDVI), soil adjusted vegetation index (SAVI), vegetation vigor index (VVI), and other spectral information to estimate ET and its parameters, which are measured on experimental watersheds with young pines and natural understory (YP), switchgrass only (SG), and young pine intercropped with switchgrass (IC). The treatment watersheds were replicated on three sites located across the Southeastern U.S. in Carteret, NC; Calhoun, MS; and Greene, AL. Despite the growth inconsistency for the SG only treatment, remote modeling estimation of ET parameters yielded an acceptable R2 > 0.70, and the ET model yielded R2 of 0.50 and a standard error of prediction of 0.94. However, ET and ET parameter model estimation for the IC performed somewhat less satisfactorily, with an R2 of 0.47, 0.59, 0.56, 0.81, and 0.57 for ET, LAI, gs, Tc, and SM, respectively, potentially due to inconsistencies in Landsat image pixel size and landuse homogeneity. Moreover, ET parameter models for the YP site performed rather poorly, with R2 = 0.28, 0.63, and 0.76 for LAI, gs, and Tc, respectively. Additionally, image analysis automation was created with Python scripting and geospatial models. The findings from this study suggest that inclusion of more spatial variability, sound data mining, ultra-high resolution imagery and advanced image processing approaches to account for potential modeling uncertainties can enhance the predictive capability of models to remotely estimate environmental parameters including ET. Radial Basis Function Network (RBFN) based models provided promising results for estimating ET and ET parameters using remotely-sensed digital information when they are prepared with advanced data mining, but it is likely that laypersons may find these models difficult to use. However, forest managers with access to neural network software can use our devised RBFN training models for estimating those forest hydrologic parameters with better accuracy.