2016 chapter
Causality-Guided Feature Selection
In Advanced Data Mining and Applications (pp. 391–405).
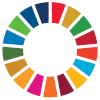
Identifying meaningful features that drive a phenomenon (response) of interest in complex systems of interconnected factors is a challenging problem. Causal discovery methods have been previously applied to estimate bounds on causal strengths of factors on a response or to identify meaningful interactions between factors in complex systems, but these approaches have been used only for inferential purposes. In contrast, we posit that interactions between factors with a potential causal association on a given response could be viable candidates not only for hypothesis generation but also for predictive modeling. In this work, we propose a causality-guided feature selection methodology that identifies factors having a potential cause-effect relationship in complex systems, and selects features by clustering them based on their causal strength with respect to the response. To this end, we estimate statistically significant causal effects on the response of factors taking part in potential causal relationships, while addressing associated technical challenges, such as multicollinearity in the data. We validate the proposed methodology for predicting response in five real-world datasets from the domain of climate science and biology. The selected features show predictive skill and consistent performance across different domains.