2021 article
DEEP TRANSFORM AND METRIC LEARNING NETWORKS
2021 IEEE INTERNATIONAL CONFERENCE ON ACOUSTICS, SPEECH AND SIGNAL PROCESSING (ICASSP 2021), pp. 2735–2739.
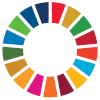
Based on its great successes in inference and denosing tasks, Dictionary Learning (DL) and its related sparse optimization formulations have garnered a lot of research interest. While most solutions have focused on single layer dictionaries, the recently improved Deep DL methods have also fallen short on a number of issues. We hence propose a novel Deep DL approach where each DL layer can be formulated and solved as a combination of one linear layer and a Recurrent Neural Network, where the RNN is flexibly regraded as a layer-associated learned metric. Our proposed work unveils new insights between the Neural Networks and Deep DL, and provides a novel, efficient and competitive approach to jointly learn the deep transforms and metrics. Extensive experiments are carried out to demonstrate that the proposed method can not only outperform existing Deep DL, but also state-of-the-art generic Convolutional Neural Networks.