2022 article
Modeling of Adaptive Receiver Performance Using Generative Adversarial Networks
IEEE 72ND ELECTRONIC COMPONENTS AND TECHNOLOGY CONFERENCE (ECTC 2022), pp. 1958–1963.
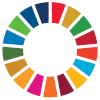
As the development of IBIS Algorithmic Modeling Interface (IBIS-AMI) models gets complex and requires time-consuming simulations, a data-driven and domain-independent approach can have tremendous value. This paper presents a data-driven approach to modeling a high-speed serializer/deserializer (SerDes) receiver through generative adversarial networks (GANs). In this work, the modeling considers multiple channels, random bitstreams, and varying decision feedback equalizer (DFE) tap values to predict an accurate bit error rate (BER) contour plot. We employ a discriminator structure that improves the training to generate a contour plot that makes it difficult to distinguish the ground truth. The generated plots’ bathtub curves strongly correlate to the ground truth bathtub curves and have a root-mean-squared error (RMSE) of 0.014, indicating a good fit.