2022 journal article
Improving Numerical Model Predicted Float Trajectories by Deep Learning
EARTH AND SPACE SCIENCE, 9(9).
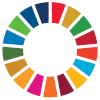
Abstract The Lagrangian tracking approach is often used in numerical modeling (NM) to simulate and predict the movement of marine particles such as plastic, oil spills, and floating wreckage. The uncertainties in NM reduce prediction accuracy as a result of the coarse temporal and spatial resolution, along with waves, winds, and currents. From 29 August to 22 December 2020, the United States Defense Advanced Research Projects Agency's Ocean of Things program deployed 422 floating drifters in the Gulf of Mexico, providing an opportunity of using the observed trajectories as ground truth to train Artificial Intelligence (AI) models to correct NM float trajectory predictions. A Regional Ocean Model System (ROMS) and AI Hybrid model was developed to implement AI to correct the ROMS‐predicted 1‐day float trajectories. The AI model is built on a convolutional neural network and Gated Recurrent Unit. The results of the ROMS‐AI Hybrid model show that 82.0% of the trajectory predictions were improved at the 24 hr, with the corresponding overall mean separation error decreasing by 11.82 km, from 20.56 to 8.74 km, which is a 57% improvement, and the error growth rate decreasing from 5.06 km per 6 hr to 1.95 km per 6 hr. The evident improvement indicates that the ROMS‐AI Hybrid model can correct the ROMS simulation to improve the 1‐day prediction of the float trajectories and shows great potential to predict the cluster of the floats.