2021 article
Non-destructive quality monitoring of 3D printed tissue scaffolds via dielectric impedance spectroscopy and supervised machine learning
49TH SME NORTH AMERICAN MANUFACTURING RESEARCH CONFERENCE (NAMRC 49, 2021), Vol. 53, pp. 636–643.
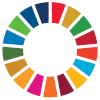
Majority of methods currently used for quality assessment of tissue engineered medical products (TEMPs) are offline and destructive in nature, which is one of the factors impeding the scale up and translation of these technologies. In this study, we investigate quality assessment of TEMP via dielectric impedance spectroscopy (DIS) and supervised machine learning (ML) as a non-destructive alternative that requires minimal human intervention. 3D printed, NaOH-treated polycaprolactone (PCL) scaffolds seeded with human adipose-derived stem cells (hASC), NIH 3T3, MG63, and human chondrocyte cells were assessed via DIS over 4 days of in vitro culture. The results showed that the cell type and duration in culture had a significant effect on the delta permittivity (Δε, an important DIS metric. Five supervised ML algorithms – K Nearest Neighbors (KNN), Logistic Regression, Random Forest Classifiers, Support Vector Machines, and artificial neural network – were then used to analyze the comprehensive structured permittivity datasets to determine their ability to discern between different cell types and culture durations. The KNN algorithm demonstrated the best accuracy (99%). The outcomes of this study demonstrate the approach of using DIS and supervised ML in conjunction for assessment of TEMPs in an automated manufacturing system.