2023 journal article
Rigorous State Evolution Analysis for Approximate Message Passing With Side Information
IEEE TRANSACTIONS ON INFORMATION THEORY, 69(6), 3989–4013.
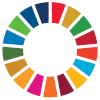
A common goal in many research areas is to reconstruct an unknown signal <inline-formula xmlns:mml="http://www.w3.org/1998/Math/MathML" xmlns:xlink="http://www.w3.org/1999/xlink"> <tex-math notation="LaTeX">$\mathbf {x}$ </tex-math></inline-formula> from noisy linear measurements. Approximate message passing (AMP) is a class of low-complexity algorithms that can be used for efficiently solving such high-dimensional regression tasks. Often, it is the case that side information (SI) is available during reconstruction, for example in online learning applications. For this reason, a novel algorithmic framework that incorporates SI into AMP, referred to as approximate message passing with side information (AMP-SI), has been recently introduced. In this work, we provide rigorous performance guarantees for AMP-SI when there are statistical dependencies between the signal and SI pairs and the entries of the measurement matrix are independent and identically distributed (i.i.d.) Gaussian. We also allow for statistical dependencies within the elements of the signal itself, by considering a flexible AMP-SI framework incorporating both separable and non-separable denoisers. The AMP-SI performance is shown to be provably tracked by a scalar iteration referred to as state evolution (SE). Moreover, we provide numerical examples that demonstrate empirically that the SE can predict the AMP-SI mean square error accurately.