2023 journal article
Classification of tree forms in aerial LiDAR point clouds using CNN for 3D tree modelling
INTERNATIONAL JOURNAL OF REMOTE SENSING, 44(22), 7156–7186.
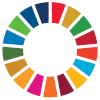
ABSTRACT Three-dimensional models of trees that correspond to the real-world forms of the trees on the ground are used in urban planning, solar power estimation, and other disciplines. Previous studies have focused on generating 3D tree models from high-density point cloud data such as Terrestrial Laser Scanning (TLS) data, which is expensive and limited to small spatial extents. However, there has been limited exploration of inexpensive solutions to model trees over large spatial extents. The goal of this study is to use widely available discrete return Airborne Laser Scanning (ALS) data along with field-captured tree photographs and Google Street View (GSV) images to develop 3D equivalents of trees over larger spatial extents. To this end, we designed a process to assign representative 3D models for individual trees in discrete return ALS point clouds. This study demonstrates the use of a Convolutional Neural Network (CNN) model and 3D models generated with Structure from Motion (SfM) for the realistic modelling of deciduous non-overlapping trees from discrete return ALS data. We classified and labelled the crown shapes of deciduous trees in a study area into four classes based on GSV images of trees. We delineated and segmented non-overlapping deciduous trees from ALS data and reduced them to 2D images using voxel point counts. Next, we trained a CNN architecture to match the 2D images to the corresponding classes observed from GSV images. For each class, we created a representative 3D tree model using field-captured circumnavigational photos of trees and SfM. To demonstrate 3D visualization using the 3D tree models, we created a 3D visualization of the trees surrounding a parking lot. The trained CNN model from this study can be used to classify non-overlapping deciduous trees from discrete return ALS data and subsequently visualize near-realistic 3D tree models of trees.