2023 journal article
Predicting and understanding residential water use with interpretable machine learning
Environmental Research Letters.
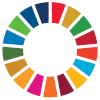
Abstract Predicting residential water use is critical to efficiently manage urban water resource systems. Simultaneously, understanding the factors driving residential water use is required to plan for future urban change and achieve effective water resource management. Current approaches examining residential water use identify the drivers of household water use through parametric or non-parametric statistical approaches. Parametric approaches have high predictive errors and lack the ability to accurately capture interactions between features but allow for easy interpretation. Non-parametric approaches have lower predictive errors and can capture non-linear feature interactions but do not allow for easy interpretation. We use non-parametric statistical models of household water use and recent advances in interpretable machine learning to understand the drivers of residential water use. Specifically, we use post-hoc interpretability methods to examine how drivers of water use interact, focusing on environmental, demographic, physical housing, and utility policy factors. We find all four categories of factors are important for estimating water use with environmental and utility policy factors playing the largest role. Additionally, we identify non-linear interactions between many variables within and across these classes. We show this approach provides both high predictive accuracy and identification of complex water use factors, offering important insight for urban water management.