2020 article
Leveraging Open-Source Tools for Collaborative Macro-energy System Modeling Efforts
DeCarolis, J. F., Jaramillo, P., Johnson, J. X., McCollum, D. L., Trutnevyte, E., Daniels, D. C., … Zhou, Y. (2020, December 16). JOULE, Vol. 4, pp. 2523–2526.
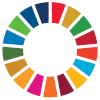
The authors are founding team members of a new effort to develop an Open Energy Outlook for the United States. The effort aims to apply best practices of policy-focused energy system modeling, ensure transparency, build a networked community, and work toward a common purpose: examining possible US energy system futures to inform energy and climate policy efforts. Individual author biographies can be found on the project website: https://openenergyoutlook.org/. The authors are founding team members of a new effort to develop an Open Energy Outlook for the United States. The effort aims to apply best practices of policy-focused energy system modeling, ensure transparency, build a networked community, and work toward a common purpose: examining possible US energy system futures to inform energy and climate policy efforts. Individual author biographies can be found on the project website: https://openenergyoutlook.org/. Many nations have committed to mitigating climate change by designing and implementing policy solutions that enable deep decarbonization of their energy systems. Due to global reliance on fossil fuels, appropriate action requires fundamental and coordinated changes in the way societies generate and use energy. Policy makers face the monumental challenge of crafting effective energy and climate policy in the face of a highly uncertain future. The stakes are high because energy infrastructure often involves large, up-front investments in long-lived assets. Macro-energy system models, which are distinguished from other energy models by their energetic, temporal, and spatial scales,1Levi P.J. Kurland S.D. Carbajales-Dale M. Weyant J.P. Brandt A.R. Benson S.M. Macro-Energy Systems: Toward a New Discipline.Joule. 2019; 3: 2282-2286Abstract Full Text Full Text PDF Scopus (29) Google Scholar provide a systematic way to examine future decarbonization pathways, evaluate technology choices, test the effects and consequences of proposed policies, and explore decisions under future uncertainty. Analyses using these models yield critical insights that inform energy and climate policymaking around the world and underpin influential reports, including the World Energy Outlook by the International Energy Agency,2International Energy AgencyWorld Energy Outlook 2019.https://www.iea.org/reports/world-energy-outlook-2019Date: 2019Google Scholar the Annual Energy Outlook by the US Energy Information Administration,3US Energy Information AdministrationAnnual Energy Outlook 2020.https://www.eia.gov/outlooks/aeo/Date: 2020Google Scholar the Special Report on Global Warming of 1.5°C by the Intergovernmental Panel on Climate Change,4Hoegh-Guldberg, O., Jacob, D., Bindi, M., Brown, S., Camilloni, I., Diedhiou, A., Djalante, R., Ebi, K., Engelbrecht, F., Guiot, J., and Hijioka, Y. (2018). Impacts of 1.5 C global warming on natural and human systems. Global warming of 1.5°C. An IPCC Special Report. https://www.ipcc.ch/sr15/.Google Scholar and many others. It is an ongoing challenge for macro-energy system modeling teams to meet the universal and unprecedented policy needs associated with climate change mitigation. We envision a paradigm shift in the process of conducting model-based analysis from single-institution modeling teams to distributed, collaborative teams, allowing access to a much wider array of disciplinary and domain expertise to inform a given analysis. While some European efforts are already moving in this direction, the potential for collaborative, model-based analysis has yet to be realized. Energy system models vary considerably in their scope and complexity, and the choice of model should always be based on the research questions driving the analysis.5DeCarolis J. Daly H. Dodds P. Keppo I. Li F. McDowall W. Pye S. Strachan N. Trutnevyte E. Usher W. Winning M. Formalizing best practice for energy system optimization modelling.Appl. Energy. 2017; 194: 184-198Crossref Scopus (159) Google Scholar Here, we focus attention on employing macro-energy system models that cover the whole energy system and are used to inform policy at scales ranging from national to global. In this broadest macro-scale context, the boundaries of the modeled systems present numerous challenges for modeling deep decarbonization pathways. First, many supply- and demand-side technologies at varying stages of development could help decarbonize energy systems. Many of these technologies are novel (e.g., direct air capture and hydrogen-based steel production), have rapidly changing costs (e.g., solar photovoltaics, lithium-ion batteries, and electrolyzers), or have location-specific attributes (e.g., heat pumps and wind farms). These qualities make the projection of technology cost and performance characteristics over the multi-decade timescale of deep decarbonization very challenging. Second, the many decision makers across the energy system, each with their own objectives and preferences, make it difficult to model technology uptake, behavioral change, and public acceptance. Third, there is a need for modeling with high spatiotemporal resolution and multiple years of weather data in order to properly represent high penetrations of renewables with energy storage and other options for flexibility, since the modeled spatial variation in resource availability and temporal variation in supply and demand can have a significant impact on results. Fourth, policy-relevant insights should account for key underlying uncertainties affecting the modeled energy system. Neglecting any of these four challenges can lead to oversimplified model representations of the energy system with misleading conclusions; yet, including them increases model complexity, data requirements, and computational burden. Resolving this tension, given available resources, is difficult. Addressing the technical challenges of modeling decarbonization pathways requires considerable coordination of effort and broad domain expertise. When the effort is centralized at a single institution, institutional and governance structures can limit its effectiveness. Energy system modeling efforts housed within a single research group can suffer from a limited breadth of expertise. At the other extreme, some of the oldest and most established energy system models have been produced by government agencies and intergovernmental organizations that have the scale to draw on deep internal expertise across the energy system, but model-based analyses produced by these organizations can be subject to political considerations that limit the range of technologies or policies they will consider. In addition, commercial modeling efforts often rely on proprietary models and data that are not available to the broader expert community or interested stakeholders and therefore result in outcomes that cannot be easily reproduced and scientifically verified. To help address these shortcomings, distributed modeling teams can utilize existing open-source models, datasets, and tools to conduct collaborative, model-based analysis. Open-source efforts in the macro-energy space have proliferated over the last decade, and the resultant models, tools, and datasets serve as an important foundation for distributed modeling efforts because they enable transparency, accessibility, and replicability among team members and with the broader modeling community. Distributed efforts focused on model-based analysis allow for the flexible arrangement of teams to conduct different macro-energy modeling exercises, with each team configured to meet project-specific research objectives. The flexible arrangement of teams, in turn, means that specific modeling efforts can include participants with different disciplinary backgrounds and domain expertise who contribute to the diversity of ideas that can be explored in the analysis. The collective consideration of those ideas better reflects the system being modeled. For example, participants with a background in public policy, public administration, or economics can assist with the formulation, execution, and interpretation of more realistic policy scenarios, informed by debates and discussions in their respective communities. Modeling teams with collectively broad expertise across a range of issues and disciplines permit a more comprehensive analysis of the technical, social, economic, and policy features of deep decarbonization pathways, which are difficult to encode in models. In fact, all team members need not write code—the purposeful inclusion of non-modelers can lead to new insights and approaches associated with the model-based analysis.6Trutnevyte E. Hirt F.L. Bauer N. Cherp A. Hawkes A. Edelenbosch O.Y. Pedde S. van Vuuren D.P. Societal transformations in models for energy and climate policy: The ambitious next step.One Earth. 2019; 1: 423-433Abstract Full Text Full Text PDF Scopus (52) Google Scholar Diverse teams participating across the full project life cycle—from the formulation of key research questions, to the decision on how to represent a particular concept quantitatively, and then to the interpretation of model results as policy-relevant insights—can more effectively capture and assimilate novel ideas compared to conventional system modeling approaches that seek feedback at the end of the project or at discrete points during the project life cycle. These insights and ideas can range widely and may include the identification and proper use of a new dataset, a new model feature that captures a system dynamic critical to the issue under analysis, or the use of more efficient algorithms or methods that improve computational performance. Modeling teams that lack the appropriate depth and breadth are less able to effectively search, select, and incorporate new ideas from the broader macro-energy idea space into the analysis. Model parsimony should also be a design objective in order to avoid needless complexity,5DeCarolis J. Daly H. Dodds P. Keppo I. Li F. McDowall W. Pye S. Strachan N. Trutnevyte E. Usher W. Winning M. Formalizing best practice for energy system optimization modelling.Appl. Energy. 2017; 194: 184-198Crossref Scopus (159) Google Scholar and thus, distributed modeling teams must judiciously filter new ideas for incorporation into the analysis. Furthermore, the expanding scope enabled by distributed teams must be balanced with limited time, funding, and computational resources. The European Union is already pioneering a distributed and collaborative approach under the €80 billion Horizon 2020 research and innovation program. Projects such as SET-NAV (https://www.set-nav.eu/), openENTRANCE (https://openentrance.eu/), SENTINEL (https://sentinel.energy/), Spine (http://www.spine-model.org/), and EMP-E (http://www.energymodellingplatform.eu/) involve large teams variously working to integrate different models into larger frameworks, solicit input from a wide array of stakeholders, and perform model-based analysis that informs European energy and climate policy. The European Union is uniquely positioned to lead such efforts, given its ambitious energy-climate policy portfolio, well-funded scientific research programs, and ambitions for pan-national integration. While many other nations and regions—including the US—cannot easily replicate the top-down European approach without a significant change in policy priorities, we nonetheless assert that it is possible for researchers to organize similar efforts from the bottom up by leveraging existing resources within the scientific community. While distributed efforts focused on model-based analysis present unique logistical challenges, they also provide the flexibility to organize teams that capture diverse domain expertise and disciplinary approaches. All of the necessary elements exist to coordinate distributed model-based analysis: open-source energy models, well-established software development tools, a wide range of collaborative communication tools, and an increasing number of publicly available datasets on which to build. First, the open energy modeling initiative (“openmod”), an active and vibrant community of energy modelers committed to open-source practices, has cataloged a large array of open-source models7Openmod InitiativeOpen Models.https://wiki.openmod-initiative.org/wiki/Open_ModelsDate: 2020Google Scholar and helped to promulgate best practice standards for model developers that include licensing, documentation, reproducibility, and user support.8DeCarolis J.F. Hunter K. Sreepathi S. The case for repeatable analysis with energy economy optimization models.Energy Econ. 2012; 34: 1845-1853Crossref Scopus (82) Google Scholar, 9Pfenninger S. Hirth L. Schlecht I. Schmid E. Wiese F. Brown T. Davis C. Gidden M. Heinrichs H. Heuberger C. Hilpert S. Opening the black box of energy modelling: Strategies and lessons learned.Energy Strategy Reviews. 2018; 19: 63-71Crossref Scopus (129) Google Scholar, 10Pfenninger S. DeCarolis J. Hirth L. Quoilin S. Staffell I. The importance of open data and software: Is energy research lagging behind?.Energy Policy. 2017; 101: 211-215Crossref Scopus (192) Google Scholar, 11Morrison R. Energy system modeling: Public transparency, scientific reproducibility, and open development.Energy Strategy Reviews. 2018; 20: 49-63Crossref Scopus (52) Google Scholar Second, many energy modelers are using modern software development tools, which enable distributed control of code and data, with changes archived in publicly accessible web repositories. Third, a variety of communication options, including traditional email, cloud-based collaboration platforms, and videoconferencing software, make it possible for distributed teams to collaborate on highly technical issues in near-real time and at low cost. These modes of communication have indeed become an increasingly familiar part of our lives given how the coronavirus disease (COVID-19) pandemic has disrupted normal meeting patterns. In addition, social media represents a particularly effective way to crowdsource new ideas and approaches from the broader stakeholder community. Fourth, the volume of available data to populate energy models has grown over time and can be used to better parameterize models. The challenge, however, is that modelers are not aware of all relevant datasets, particularly those curated outside of the energy modeling community, nor do they always understand the underlying assumptions and limitations. Diversity in expertise among the modeling team can help ensure the proper identification and use of such datasets. In the long run, by using open-source tools and drawing on the expertise of non-modelers who are typically disconnected from the modeling process, distributed modeling teams may counteract the “incumbency advantage” of “long-lived and dominant” energy models12Strachan N. Fais B. Daly H. Reinventing the energy modelling–policy interface.Nat. Energy. 2016; 1: 1-3Crossref Google Scholar by helping redefine the way energy models operate. We view this approach as a critical element in the reinvention of the modeling-policy interface.12Strachan N. Fais B. Daly H. Reinventing the energy modelling–policy interface.Nat. Energy. 2016; 1: 1-3Crossref Google Scholar As with any new approach, there will be attendant challenges. Macro-energy modeling efforts face the same funding and coordination challenges confronted by other large scientific endeavors. Funding challenges are more logistically difficult with teams spanning multiple institutions. There is no single solution: financial arrangements will necessarily be a product of the funding agency, team composition, and objectives of the analysis. While there may be circumstances where funding can be equitably distributed among all participants, there might be other times when one or two lead organization(s) take the bulk of the responsibility, with smaller support grants and in-kind contributions from other members of the distributed team. Furthermore, funding need not always be a requirement for participation: limited but strategic input from a broad constellation of team members delivered at the right time in the process can have a large, positive impact on the direction of the project. While the Stanford Energy Modeling Forum (https://emf.stanford.edu/) is focused on inter-model comparison, its long-term success demonstrates that participants are willing to contribute their time, often without financial compensation, in return for the opportunity to collaborate with others and produce new scholarly research. Another challenge is the incentive structure within academia. It takes significant upfront effort to establish a common language and align project goals among team members from different academic disciplines. In addition, receiving credit for work completed is an important aspect of scholarly work. Credit often takes the form of co-authorship on reports and journal articles, and it is important to track the contributions of team members to ensure their efforts are recognized in an appropriate way, commensurate with their own institutional and disciplinary incentive structures. Furthermore, academic institutions should formally recognize the effort required to develop the open-source models, tools, and datasets that underpin the model-based analysis. The CRediT taxonomy, used by this publisher (https://www.cell.com/pb/assets/raw/shared/guidelines/CRediT-taxonomy.pdf), provides an excellent way to track the various contributions to distributed macro-energy modeling efforts. New modeling efforts that leverage these emerging opportunities can fulfill a unique niche within the global energy modeling community. We have begun to see the benefits of such an approach in our own effort to develop an Open Energy Outlook for the US (https://openenergyoutlook.org/). In addition to using an open-source modeling platform to perform the analysis (https://temoacloud.com/), we have established an interdisciplinary and inter-sectoral team of experts who are working collaboratively on the project with a unified vision. Our international team involves a number of experts drawn from academia, non-profits, and government labs and includes both experienced macro-energy system modelers and domain experts. Funding is distributed across two institutions that have primary responsibility for the deliverables, while participants from the remaining 20+ institutions make in-kind contributions of their time to the effort. Our project has a fraction of the funding associated with the large European efforts referenced above, and thus relies heavily on our collective interest in the project objectives and the opportunity to collaboratively produce scholarly work. Because participants are already working in related areas, they are able to leverage ongoing research activities and resources for this project. Our current team is meant to be a starting point for this long-term effort. Just as open-source tools foster collaborative development, democratization of the team building process can ensure a greater diversity of perspectives and make the effort more adaptable to new challenges. To this end, we are currently working on a formal and open nomination process for team membership. In addition, we are building a broader network of contributors to the project, and have sought input through a variety of online outlets, including social media, virtual workshops, and mailing lists. While still in the early stages, the project has already benefited from the diverse perspectives of the participants. For example, the electricity experts have pushed for a novel approach to increase the model’s temporal resolution while maintaining computational tractability and also identified opportunities to leverage existing open-source tools (https://github.com/gschivley/PowerGenome) and datasets (https://github.com/catalyst-cooperative/pudl). Likewise, the building experts are pushing the project to consider building thermodynamics more explicitly in order to better represent building thermal performance. The value here is bidirectional: systems modelers gain more familiarity with tools and data within particular sectors, while domain experts gain a better understanding of how their expertise can influence long-term energy scenarios. If done well, such an approach allows us to rethink and redefine common modeling approaches, potentially leading to innovative methods that result in new insights that are rigorously grounded by careful consideration of how the energy system—and all its myriad connections and feedbacks—is modeled. We would like to thank the Alfred P. Sloan Foundation for supporting this work. We also thank the two anonymous reviewers whose detailed and insightful feedback significantly strengthened the manuscript. Leveraging Open-Source Tools for Collaborative Macro-energy System Modeling EffortsDeCarolis et al.JouleFebruary 17, 2021In Brief(Joule 4, 2523–2531; December 16, 2020) Full-Text PDF Open Access