2021 journal article
SEAGLE: A Scalable Exact Algorithm for Large-Scale Set-Based Gene-Environment Interaction Tests in Biobank Data
FRONTIERS IN GENETICS, 12.
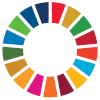
The explosion of biobank data offers unprecedented opportunities for gene-environment interaction (GxE) studies of complex diseases because of the large sample sizes and the rich collection in genetic and non-genetic information. However, the extremely large sample size also introduces new computational challenges in G×E assessment, especially for set-based G×E variance component (VC) tests, which are a widely used strategy to boost overall G×E signals and to evaluate the joint G×E effect of multiple variants from a biologically meaningful unit (e.g., gene). In this work, we focus on continuous traits and present SEAGLE, a Scalable Exact AlGorithm for Large-scale set-based G×E tests, to permit G×E VC tests for biobank-scale data. SEAGLE employs modern matrix computations to calculate the test statistic and p-value of the GxE VC test in a computationally efficient fashion, without imposing additional assumptions or relying on approximations. SEAGLE can easily accommodate sample sizes in the order of 105, is implementable on standard laptops, and does not require specialized computing equipment. We demonstrate the performance of SEAGLE using extensive simulations. We illustrate its utility by conducting genome-wide gene-based G×E analysis on the Taiwan Biobank data to explore the interaction of gene and physical activity status on body mass index.