Works (46)
2024 journal article
A joint reconstruction and model selection approach for large-scale linear inverse modeling (msHyBR v2)
GEOSCIENTIFIC MODEL DEVELOPMENT, 17(23), 8853–8872.
2024 journal article
Efficient iterative methods for hyperparameter estimation in large-scale linear inverse problems
ADVANCES IN COMPUTATIONAL MATHEMATICS, 50(6).
2023 journal article
A COMPUTATIONAL FRAMEWORK FOR EDGE-PRESERVING REGULARIZATION IN DYNAMIC INVERSE PROBLEMS
ELECTRONIC TRANSACTIONS ON NUMERICAL ANALYSIS, 58, 486–516.
2023 journal article
EFFICIENT ALGORITHMS FOR BAYESIAN INVERSE PROBLEMS WITH WHITTLE--MATERN PRIORS
SIAM JOURNAL ON SCIENTIFIC COMPUTING, 46(2), S176–S198.
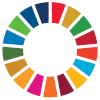
2023 journal article
HYBRID PROJECTION METHODS FOR SOLUTION DECOMPOSITION IN LARGE-SCALE BAYESIAN INVERSE PROBLEMS\ast
SIAM JOURNAL ON SCIENTIFIC COMPUTING, 46(2), S97–S119.
2023 journal article
MONTE CARLO METHODS FOR ESTIMATING THE DIAGONAL OF A REAL SYMMETRIC MATRIX
SIAM JOURNAL ON MATRIX ANALYSIS AND APPLICATIONS, 44(1), 240–269.
2023 journal article
RANDOMIZED ALGORITHMS FOR ROUNDING IN THE TENSOR-TRAIN FORMAT
SIAM JOURNAL ON SCIENTIFIC COMPUTING, 45(1), A74–A95.
2023 journal article
Randomized reduced basis methods for parameterized fractional elliptic PDEs
FINITE ELEMENTS IN ANALYSIS AND DESIGN, 227.
2023 journal article
Tensor-based flow reconstruction from optimally located sensor measurements
JOURNAL OF FLUID MECHANICS, 962.
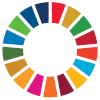
2022 journal article
Computationally efficient methods for large-scale atmospheric inverse modeling
GEOSCIENTIFIC MODEL DEVELOPMENT, 15(14), 5547–5565.
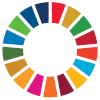
2022 journal article
Efficient randomized tensor-based algorithms for function approximation and low-rank kernel interactions
ADVANCES IN COMPUTATIONAL MATHEMATICS, 48(5).
2022 journal article
Kryging: geostatistical analysis of large-scale datasets using Krylov subspace methods
STATISTICS AND COMPUTING, 32(5).
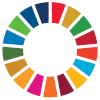
2022 journal article
STRUCTURED MATRIX APPROXIMATIONS VIA TENSOR DECOMPOSITIONS
SIAM JOURNAL ON MATRIX ANALYSIS AND APPLICATIONS, 43(4), 1599–1626.
2021 journal article
EFFICIENT ALGORITHMS FOR EIGENSYSTEM REALIZATION USING RANDOMIZED SVD
SIAM JOURNAL ON MATRIX ANALYSIS AND APPLICATIONS, 42(2), 1045–1072.
2021 journal article
MONTE CARLO ESTIMATORS FOR THE SCHATTEN p-NORM OF SYMMETRIC POSITIVE SEMIDEFINITE MATRICES
ELECTRONIC TRANSACTIONS ON NUMERICAL ANALYSIS, 55, 213–241.
2021 journal article
Randomized algorithms for generalized singular value decomposition with application to sensitivity analysis
NUMERICAL LINEAR ALGEBRA WITH APPLICATIONS, 28(4).
2021 journal article
Randomized approaches to accelerate MCMC algorithms for Bayesian inverse problems
JOURNAL OF COMPUTATIONAL PHYSICS, 440.
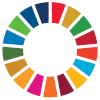
2020 journal article
Efficient Krylov subspace methods for uncertainty quantification in large Bayesian linear inverse problems
NUMERICAL LINEAR ALGEBRA WITH APPLICATIONS, 27(5).
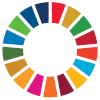
2020 journal article
Geostatistical inverse modeling with very large datasets: an example from the Orbiting Carbon Observatory 2 (OCO-2) satellite
GEOSCIENTIFIC MODEL DEVELOPMENT, 13(3), 1771–1785.
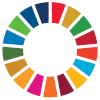
2020 journal article
RANDOMIZATION AND REWEIGHTED l(1)-MINIMIZATION FOR A-OPTIMAL DESIGN OF LINEAR INVERSE PROBLEMS
SIAM JOURNAL ON SCIENTIFIC COMPUTING, 42(3), A1714–A1740.
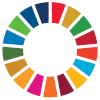
2020 journal article
Randomized Algorithms for Low-Rank Tensor Decompositions in the Tucker Format
SIAM JOURNAL ON MATHEMATICS OF DATA SCIENCE, 2(1), 189–215.
2020 journal article
Randomized Discrete Empirical Interpolation Method for Nonlinear Model Reduction
SIAM Journal on Scientific Computing.
2019 journal article
Efficient Marginalization-Based MCMC Methods for Hierarchical Bayesian Inverse Problems
SIAM-ASA JOURNAL ON UNCERTAINTY QUANTIFICATION, 7(3), 1105–1131.
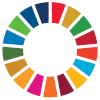
2019 journal article
RANDOMIZED SUBSPACE ITERATION: ANALYSIS OF CANONICAL ANGLES AND UNITARILY INVARIANT NORMS
SIAM JOURNAL ON MATRIX ANALYSIS AND APPLICATIONS, 40(1), 23–48.
2018 journal article
A randomized tensor singular value decomposition based on the t-product
NUMERICAL LINEAR ALGEBRA WITH APPLICATIONS, 25(5).
2018 journal article
EFFICIENT D-OPTIMAL DESIGN OF EXPERIMENTS FOR INFINITE-DIMENSIONAL BAYESIAN LINEAR INVERSE PROBLEMS
SIAM JOURNAL ON SCIENTIFIC COMPUTING, 40(5), A2956–A2985.
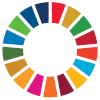
2018 journal article
Goal-oriented optimal design of experiments for large-scale Bayesian linear inverse problems
INVERSE PROBLEMS, 34(9).
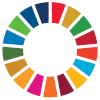
2018 journal article
Going Off the Grid: Iterative Model Selection for Biclustered Matrix Completion
JOURNAL OF COMPUTATIONAL AND GRAPHICAL STATISTICS, 28(1), 36–47.
2018 journal article
Low-Rank Independence Samplers in Hierarchical Bayesian Inverse Problems
SIAM-ASA JOURNAL ON UNCERTAINTY QUANTIFICATION, 6(3), 1076–1100.
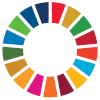
2018 journal article
THE DISCRETE EMPIRICAL INTERPOLATION METHOD: CANONICAL STRUCTURE AND FORMULATION IN WEIGHTED INNER PRODUCT SPACES
SIAM JOURNAL ON MATRIX ANALYSIS AND APPLICATIONS, 39(3), 1152–1180.
2017 journal article
Efficient generalized Golub-Kahan based methods for dynamic inverse problems
Inverse Problems, 34(2).
Contributors: J. Chung *, n , M. Brown* & E. Westman *
2017 journal article
GENERALIZED HYBRID ITERATIVE METHODS FOR LARGE-SCALE BAYESIAN INVERSE PROBLEMS
SIAM JOURNAL ON SCIENTIFIC COMPUTING, 39(5), S24–S46.
2017 journal article
MULTIPRECONDITIONED GMRES FOR SHIFTED SYSTEMS
SIAM JOURNAL ON SCIENTIFIC COMPUTING, 39(5), S222–S247.
2017 journal article
Randomized matrix-free trace and log-determinant estimators
NUMERISCHE MATHEMATIK, 137(2), 353–395.
Contributors: , A. Alexanderian n & I. Ipsen n n
2016 journal article
HOID: HIGHER ORDER INTERPOLATORY DECOMPOSITION FOR TENSORS BASED ON TUCKER REPRESENTATION
SIAM JOURNAL ON MATRIX ANALYSIS AND APPLICATIONS, 37(3), 1223–1249.
2015 conference paper
3D parameter reconstruction in hyperspectral diffuse optical tomography
Progress in Biomedical Optics and Imaging - Proceedings of SPIE, 9319.
Contributors: , N. Krishnamurthy*, P. Anderson *, J. Kainerstorfer *, A. Sassaroli *, E. Miller *, S. Fantini *, M. Kilmer * *
2015 journal article
A fast algorithm for parabolic PDE-based inverse problems based on Laplace transforms and flexible Krylov solvers
Journal of Computational Physics, 299, 940–954.
Contributors: T. Bakhos*, * & P. Kitanidis *
2015 journal article
Fast Kalman filter using hierarchical matrices and a low-rank perturbative approach
Inverse Problems, 31(1).
Contributors: , E. Miller * & P. Kitanidis * *
2015 journal article
Fast algorithms for hyperspectral diffuse optical tomography
SIAM Journal on Scientific Computing, 37(5), B712–B743.
2015 journal article
Fast computation of uncertainty quantification measures in the geostatistical approach to solve inverse problems
Advances in Water Resources, 82, 124–138.
2015 journal article
Randomized algorithms for generalized Hermitian eigenvalue problems with application to computing Karhunen-Loève expansion
Numerical Linear Algebra with Applications, 23(2), 314–339.
Contributors: , J. Lee * & P. Kitanidis * *
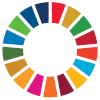
2014 conference paper
A fast Kalman filter for time-lapse electrical resistivity tomography
International Geoscience and Remote Sensing Symposium (IGARSS), 3152–3155.
Contributors: , E. Miller * & P. Kitandis* *
2013 journal article
A flexible krylov solver for shifted systemswith application to oscillatory hydraulic tomography
SIAM Journal on Scientific Computing, 35(6).
2013 article
Fast Algorithms for Bayesian Inversion
Computational Challenges in the Geosciences, pp. 101–142.
2012 journal article
Application of hierarchical matrices to linear inverse problems in geostatistics | Application des matrices hiérarchiques aux problèmes d'inversion linéaire en géostatistique
Oil and Gas Science and Technology, 67(5), 857–875.
2012 journal article
Efficient methods for large-scale linear inversion using a geostatistical approach
Water Resources Research, 48(5).
Contributors: & P. Kitanidis * *
Employment
Updated: October 30th, 2020 16:09
2020 - present
2015 - 2020
2013 - 2015
Education
Updated: February 14th, 2018 12:03
2008 - 2013
2007 - 2010
2003 - 2007
Funding History
Funding history based on the linked ORCID record. Updated: October 15th, 2019 14:35