Dario Del Giudice
Quantitative risk assessment, Parsimonious Process-Based Modeling, Bayesian Statistics, Uncertainty Quantification
Dr. Dario Del Giudice is conducting his research at NCSU, after moving from Stanford where he was working at the Carnegie Institution for Science. He is interested in understanding and predicting the impacts of climate variability and human pressures on the quantity and quality of water resources. In his research at the interface of catchment hydrology and surface water pollution he uniquely combines process-based models, stochastic methods for uncertainty quantification, and statistical inference. Dr. Del Giudice is currently focusing on the complex issue of fertilizer running off agricultural watersheds into surface waters and fostering eutrophication and dead zones in critical systems such as the Great Lakes and the Gulf of Mexico. His goal is to identify the key hydroclimatic and land-management factors responsible for lacustrine, estuarine, and coastal hypoxia and predict how the quality of these aquatic ecosystems will respond to changing patterns in precipitation and temperature. Dr. Del Giudice earned his PhD degree from the Swiss Federal Institute of Technology in Zurich. During his doctorate, he has worked on improving inference of catchment properties, rainfall estimation, and predictions of hydrological and contaminant transport dynamics. In particular, he has developed advanced Bayesian methods to assimilate runoff data and quantify predictive uncertainty coming from errors in model input (related to precipitation) and structure (related to system oversimplifications).
Works (23)
2023 article
SmitomB/Internal_Phosphorus_Loading_Model: Characterizing and Projecting Internal Phosphorus Loading through Bayesian Mass-balance Modeling
(2023, December 23). Zenodo.
Contributors: S. Borah, D. Giudice, M. Aupperle & D. Obenour
2023 article
SmitomB/Internal_Phosphorus_Loading_Model: Characterizing and Projecting Internal Phosphorus Loading through Bayesian Mass-balance Modeling
(2023, December 23). Zenodo.
Contributors: S. Borah, D. Giudice, M. Aupperle & D. Obenour
2022 journal article
Temporally resolved coastal hypoxia forecasting and uncertainty assessment via Bayesian mechanistic modeling
HYDROLOGY AND EARTH SYSTEM SCIENCES, 26(4), 1131–1143.
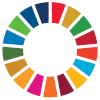
2021 journal article
Simulating algal dynamics within a Bayesian framework to evaluate controls on estuary productivity
ECOLOGICAL MODELLING, 447.
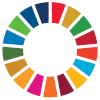
2020 journal article
Accounting for erroneous model structures in biokinetic process models
RELIABILITY ENGINEERING & SYSTEM SAFETY, 203.
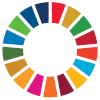
2020 journal article
Elucidating controls on cyanobacteria bloom timing and intensity via Bayesian mechanistic modeling
SCIENCE OF THE TOTAL ENVIRONMENT, 755.
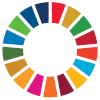
2020 journal article
Exploring nutrient and light limitation of algal production in a shallow turbid reservoir
ENVIRONMENTAL POLLUTION, 269.
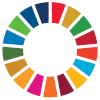
2019 journal article
A space-time geostatistical model for probabilistic estimation of harmful algal bloom biomass and areal extent
Science of The Total Environment, 8, 133776.
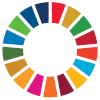
2019 journal article
Bayesian mechanistic modeling characterizes Gulf of Mexico hypoxia: 1968-2016 and future scenarios
ECOLOGICAL APPLICATIONS, 30(2).
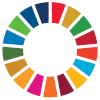
2019 journal article
Modeling biophysical controls on hypoxia in a shallow estuary using a Bayesian mechanistic approach
Environmental Modelling & Software, 7.
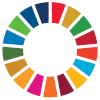
2018 journal article
Accelerating Bayesian inference in hydrological modeling with a mechanistic emulator
Environmental Modelling & Software, 7.
2018 journal article
Long-term phosphorus loading and springtime temperatures explain interannual variability of hypoxia in a large temperate lake
Environmental Science & Technology, 1.
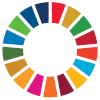
2018 journal article
On the practical usefulness of least squares for assessing uncertainty in hydrologic and water quality predictions
ENVIRONMENTAL MODELLING & SOFTWARE, 105, 286–295.
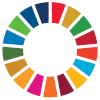
2017 journal article
2016 journal article
Describing the catchment-averaged precipitation as a stochastic process improves parameter and input estimation
Water Resour. Res., 3, n/a-n/a.
2016 journal article
2015 journal article
Comparison of two stochastic techniques for reliable urban runoff prediction by modeling systematic errors
Water Resour. Res., 5, n/a-n/a.
2015 journal article
The value of streamflow data in improving TSS predictions - Bayesian multi-objective calibration
Journal of Hydrology, 9, 241–254.
Contributors: A. Sikorska*, * , K. Banasik * & J. Rieckermann *
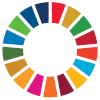
2014 journal article
Model bias and complexity – Understanding the effects of structural deficits and input errors on runoff predictions
Environmental Modelling & Software, 64(1), 205–214.
Contributors: , P. Reichert *, V. Bareš *, C. Albert * & J. Rieckermann * *
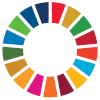
2013 journal article
Dynamic time warping improves sewer flow monitoring
Water Research, 47(11), 3803–3816.
Contributors: D. Dürrenmatt*, * & J. Rieckermann *
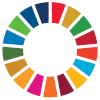
2013 journal article
Improving uncertainty estimation in urban hydrological modeling by statistically describing bias
Hydrology and Earth System Sciences, 17(10), 4209–4225.
Contributors: , M. Honti *, A. Scheidegger *, C. Albert *, P. Reichert * & J. Rieckermann * *
2012 journal article
Modeling of facade leaching in urban catchments
Water Resources Research, 48(12).
Contributors: S. Coutu*, * , L. Rossi * & D. Barry *
2012 journal article
Parsimonious hydrological modeling of urban sewer and river catchments
Journal of Hydrology, 464-465, 477–484.
Contributors: S. Coutu*, * , L. Rossi* & D. Barry *
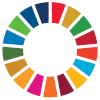