Farah Raed Hussein Alsafadi
Is this your profile?
Claim your Citation Index profile in order to display more information about you and gain access to Libraries services. Just create or connect your ORCID iD.
Create or connect your ORCID iD
Works (7)
2025 journal article
Data-driven prediction and uncertainty quantification of PWR crud-induced power shift using convolutional neural networks
ENERGY, 316.
2024 journal article
ARTISANS-Artificial Intelligence for Simulation of Advanced Nuclear Systems for Nuclear Fission Technology
NUCLEAR ENGINEERING AND DESIGN, 423.
2024 article
Development of Whole System Digital Twins for Advanced Reactors: Leveraging Graph Neural Networks and SAM Simulations
Liu, Y., Alsafadi, F., Mui, T., O'Grady, D., & Hu, R. (2024, October 13). NUCLEAR TECHNOLOGY.
2023 journal article
Deep generative modeling-based data augmentation with demonstration using the BFBT benchmark void fraction datasets
NUCLEAR ENGINEERING AND DESIGN, 415.
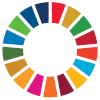
2022 journal article
Effect of mesh refinement on the solution of the inverse uncertainty quantification problem for transient physics
PROGRESS IN NUCLEAR ENERGY, 152.
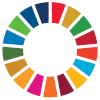
2021 journal article
A comprehensive survey of inverse uncertainty quantification of physical model parameters in nuclear system thermal-hydraulics codes
NUCLEAR ENGINEERING AND DESIGN, 384.
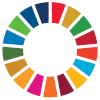
2021 journal article
Towards improving the predictive capability of computer simulations by integrating inverse Uncertainty Quantification and quantitative validation with Bayesian hypothesis testing
NUCLEAR ENGINEERING AND DESIGN, 383.
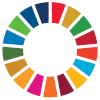