Gangshan Jing
multi-agent systems, formation control, reinforcement learning, network localization
Works (6)
2022 journal article
Fuel-Optimal Guidance for End-to-End Human-Mars Entry, Powered-Descent, and Landing Mission
IEEE TRANSACTIONS ON AEROSPACE AND ELECTRONIC SYSTEMS, 58(4), 2837–2854.
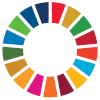
2022 journal article
Learning Distributed Stabilizing Controllers for Multi-Agent Systems
IEEE CONTROL SYSTEMS LETTERS, 6, 301–306.
2021 article
Decomposability and Parallel Computation of Multi-Agent LQR
2021 AMERICAN CONTROL CONFERENCE (ACC), pp. 4527–4532.
2021 article
Local Shape-Preserving Formation Maneuver Control of Multi-agent Systems: From 2D to 3D
2021 60TH IEEE CONFERENCE ON DECISION AND CONTROL (CDC), pp. 6251–6257.
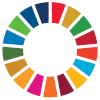
2021 journal article
Model-Free Optimal Control of Linear Multiagent Systems via Decomposition and Hierarchical Approximation
IEEE TRANSACTIONS ON CONTROL OF NETWORK SYSTEMS, 8(3), 1069–1081.
2020 journal article
Model-Free Reinforcement Learning of Minimal-Cost Variance Control
IEEE CONTROL SYSTEMS LETTERS, 4(4), 916–921.