Jingzhu He
Works (6)
2021 article
Mining Anomalies in Subspaces of High-Dimensional Time Series for Financial Transactional Data
MACHINE LEARNING AND KNOWLEDGE DISCOVERY IN DATABASES, ECML PKDD 2021: APPLIED DATA SCIENCE TRACK, PT IV, Vol. 12978, pp. 19–36.
2020 article
Self-Patch: Beyond Patch Tuesday for Containerized Applications
2020 IEEE INTERNATIONAL CONFERENCE ON AUTONOMIC COMPUTING AND SELF-ORGANIZING SYSTEMS (ACSOS 2020), pp. 21–27.
2019 article
A Study on Container Vulnerability Exploit Detection
2019 IEEE INTERNATIONAL CONFERENCE ON CLOUD ENGINEERING (IC2E), pp. 121–127.
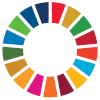
2019 article
TFix: Automatic Timeout Bug Fixing in Production Server Systems
2019 39TH IEEE INTERNATIONAL CONFERENCE ON DISTRIBUTED COMPUTING SYSTEMS (ICDCS 2019), pp. 612–623.
2018 article
DScope: Detecting Real-World Data Corruption Hang Bugs in Cloud Server Systems
PROCEEDINGS OF THE 2018 ACM SYMPOSIUM ON CLOUD COMPUTING (SOCC '18), pp. 313–325.
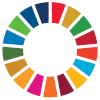
2018 article
TScope: Automatic Timeout Bug Identification for Server Systems
15TH IEEE INTERNATIONAL CONFERENCE ON AUTONOMIC COMPUTING (ICAC 2018), pp. 1–10.