Is this your profile?
Claim your Citation Index profile in order to display more information about you and gain access to Libraries services. Just create or connect your ORCID iD.
Create or connect your ORCID iD
Works (7)
2024 article
Protein-Protein Interaction Networks Derived from Classical and Machine Learning-Based Natural Language Processing Tools
Degnan, D. J., Strauch, C. W., Obiri, M. Y., Vonkaenel, E. D., Kim, G. S., Kershaw, J. D., … Bramer, L. M. (2024, November 11). JOURNAL OF PROTEOME RESEARCH.
2022 journal article
A Comparison of Infectious Disease Forecasting Methods across Locations, Diseases, and Time
PATHOGENS, 11(2).
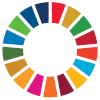
2022 journal article
Dynamic logistic regression and variable selection: Forecasting and contextualizing civil unrest
INTERNATIONAL JOURNAL OF FORECASTING, 38(2), 648–661.
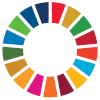
2022 journal article
Predicting infectious disease for biopreparedness and response: A systematic review of machine learning and deep learning approaches
ONE HEALTH, 15.
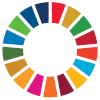
2021 article
Estimating basis functions in massive fields under the spatial mixed effects model
Pazdernik, K., & Maitra, R. (2021, July 31). STATISTICAL ANALYSIS AND DATA MINING.
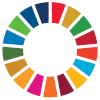
2020 journal article
Microstructural classification of unirradiated LiAlO2 pellets by deep learning methods
COMPUTATIONAL MATERIALS SCIENCE, 181.
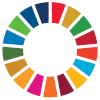
2018 journal article
Twitter geolocation: A hybrid approach
ACM Transactions on Knowledge Discovery from Data, 12(3).