Works (5)
2024 journal article
Risk score models for urinary tract infection hospitalization
PLOS ONE, 19(6).
Ed(s): G. Villavicencio
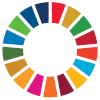
2022 article
Improved feature selection with simulation optimization
Shashaani, S., & Vahdat, K. (2022, May 30). OPTIMIZATION AND ENGINEERING, Vol. 5.
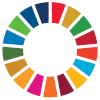
2022 conference paper
Personalized Predictions for Unplanned Urinary Tract Infection Hospitalizations with Hierarchical Clustering
Springer Proceedings in Business and Economics, 453–465.
2021 conference paper
Non-Parametric Uncertainty Bias and Variance Estimation via Nested Bootstrapping and Influence Functions
2021 Winter Simulation Conference (WSC).
2020 article
SIMULATION OPTIMIZATION BASED FEATURE SELECTION, A STUDY ON DATA-DRIVEN OPTIMIZATION WITH INPUT UNCERTAINTY
2020 WINTER SIMULATION CONFERENCE (WSC), pp. 2149–2160.
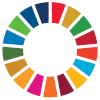
Employment
Updated: April 26th, 2022 10:14
2018 - 2023