Max Gordon
Is this your profile?
Claim your Citation Index profile in order to display more information about you and gain access to Libraries services. Just create or connect your ORCID iD.
Create or connect your ORCID iD
Works (4)
2024 article
Global genotype by environment prediction competition reveals that diverse modeling strategies can deliver satisfactory maize yield estimates
Washburn, J. D., Varela, J. I., Xavier, A., Chen, Q., Ertl, D., Gage, J. L., … Leon, N. (2024, December 27). (M. Sillanpää, Ed.). GENETICS, Vol. 12.
Ed(s): M. Sillanpää
2022 review
Compositionality, sparsity, spurious heterogeneity, and other data-driven challenges for machine learning algorithms within plant microbiome studies
[Review of ]. CURRENT OPINION IN PLANT BIOLOGY, 71.
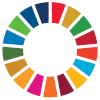
2022 journal article
FER and LecRK show haplotype-dependent cold-responsiveness and mediate freezing tolerance in Lotus japonicus
PLANT PHYSIOLOGY, 191(2), 1138–1152.
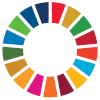
2020 journal article
Gene Regulatory Network Inference: Connecting Plant Biology and Mathematical Modeling
Frontiers in Genetics, 11.
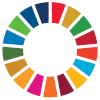