Ranga Raju Vatsavai
GeoSpatial AI, Machine Learning, Data Mining, Spatial and Temporal, Remote Sensing, Image and Video Understanding, Computer Vision, Crop Biomass Monitoring, Settlement Mapping, HPC, Spatial Databases, Climate Smart Agriculture and Forestry
Works (48)
2024 article
Geospatial Foundation Models: Recent Advances and Applications
PROCEEDINGS OF THE 12TH ACM SIGSPATIAL INTERNATIONAL WORKSHOP ON ANALYTICS FOR BIG GEOSPATIAL DATA, BIGSPATIAL 2024, pp. 30–33.
2024 article
Multi-spectral Gradient Residual Network for Haze Removal in Multi-sensor Remote Sensing Imagery
MACHINE LEARNING AND KNOWLEDGE DISCOVERY IN DATABASES-APPLIED DATA SCIENCE TRACK, PT X, ECML PKDD 2024, Vol. 14950, pp. 413–428.
2023 article
Cloud Imputation for Multi-sensor Remote Sensing Imagery with Style Transfer
MACHINE LEARNING AND KNOWLEDGE DISCOVERY IN DATABASES: APPLIED DATA SCIENCE AND DEMO TRACK, ECML PKDD 2023, PT VII, Vol. 14175, pp. 37–53.
2023 article
Context Retrieval via Normalized Contextual Latent Interaction for Conversational Agent
2023 23RD IEEE INTERNATIONAL CONFERENCE ON DATA MINING WORKSHOPS, ICDMW 2023, pp. 1543–1550.
2023 article
Harmonization-guided deep residual network for imputing under clouds with multi-sensor satellite imagery
PROCEEDINGS OF 2023 18TH INTERNATIONAL SYMPOSIUM ON SPATIAL AND TEMPORAL DATA, SSTD 2023, pp. 151–160.
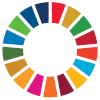
2023 article
NOVEL DEEP LEARNING FRAMEWORK FOR IMPUTING HOLES IN ORTHORECTIFIED VHR IMAGES
IGARSS 2023 - 2023 IEEE INTERNATIONAL GEOSCIENCE AND REMOTE SENSING SYMPOSIUM, pp. 5158–5161.
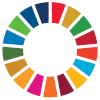
2023 article
Persona-Coded Poly-Encoder: Persona-Guided Multi-Stream Conversational Sentence Scoring
2023 IEEE 35TH INTERNATIONAL CONFERENCE ON TOOLS WITH ARTIFICIAL INTELLIGENCE, ICTAI, pp. 250–257.
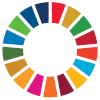
2023 conference paper
Q-learning Based Simulation Tool for Studying Effectiveness of Dynamic Application of Fertilizer on Crop Productivity
Mei, Z., Vatsavai, R., & Chirkova, R. (2023, November 13).
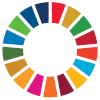
2023 journal article
Remote Sensing Based Crop Type Classification Via Deep Transfer Learning
IEEE JOURNAL OF SELECTED TOPICS IN APPLIED EARTH OBSERVATIONS AND REMOTE SENSING, 16, 4699–4712.
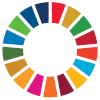
2022 journal article
A Survey of Single-Scene Video Anomaly Detection
IEEE TRANSACTIONS ON PATTERN ANALYSIS AND MACHINE INTELLIGENCE, 44(5), 2293–2312.
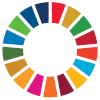
2022 article
Deep Residual Network with Multi-Image Attention for Imputing Under Clouds in Satellite Imagery
2022 26TH INTERNATIONAL CONFERENCE ON PATTERN RECOGNITION (ICPR), pp. 643–649.
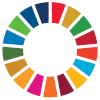
2022 article
Multi-stream Deep Residual Network for Cloud Imputation Using Multi-resolution Remote Sensing Imagery
2022 21ST IEEE INTERNATIONAL CONFERENCE ON MACHINE LEARNING AND APPLICATIONS, ICMLA, pp. 97–104.
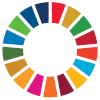
2022 article
Persona-Based Conversational AI: State of the Art and Challenges
2022 IEEE INTERNATIONAL CONFERENCE ON DATA MINING WORKSHOPS, ICDMW, pp. 993–1001.
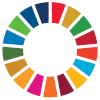
2022 article
Real-Time Change Detection At the Edge
2022 21ST IEEE INTERNATIONAL CONFERENCE ON MACHINE LEARNING AND APPLICATIONS, ICMLA, pp. 776–781.
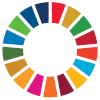
2021 article
A Scalable System for Searching Large-scale Multi-sensor Remote Sensing Image Collections
2021 IEEE INTERNATIONAL CONFERENCE ON BIG DATA (BIG DATA), pp. 3780–3783.
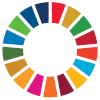
2021 article
Local Clustering with Mean Teacher for Semi-supervised learning
2020 25TH INTERNATIONAL CONFERENCE ON PATTERN RECOGNITION (ICPR), pp. 6243–6250.
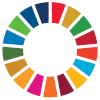
2021 journal article
Perceptual metric learning for video anomaly detection
MACHINE VISION AND APPLICATIONS, 32(3).
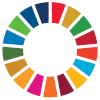
2019 journal article
Anomalous cluster detection in spatiotemporal meteorological fields
STATISTICAL ANALYSIS AND DATA MINING, 12(2), 88–100.
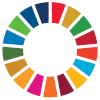
2018 article
Deformable Part Models for Complex Object Detection in Remote Sensing Imagery
BIGSPATIAL 2018: PROCEEDINGS OF THE 7TH ACM SIGSPATIAL INTERNATIONAL WORKSHOP ON ANALYTICS FOR BIG GEOSPATIAL DATA (BIGSPATIAL-2018), pp. 57–62.
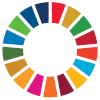
2018 article
FUTURES-DPE: Towards Dynamic Provisioning and Execution of Geosimulations in HPC environments
26TH ACM SIGSPATIAL INTERNATIONAL CONFERENCE ON ADVANCES IN GEOGRAPHIC INFORMATION SYSTEMS (ACM SIGSPATIAL GIS 2018), pp. 464–467.
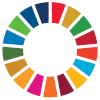
2018 article
Machine Learning Approaches for Slum Detection Using Very High Resolution Satellite Images
2018 18TH IEEE INTERNATIONAL CONFERENCE ON DATA MINING WORKSHOPS (ICDMW), pp. 1397–1404.
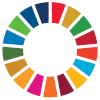
2018 journal article
Real-Time Energy Audit of Built Environments: Simultaneous Localization and Thermal Mapping
JOURNAL OF INFRASTRUCTURE SYSTEMS, 24(3).
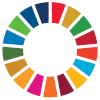
2017 conference paper
Hierarchical change detection framework for biomass monitoring
2017 ieee international geoscience and remote sensing symposium (igarss), 620–623.
2017 journal article
High performance GPU computing based approaches for oil spill detection from multi-temporal remote sensing data
REMOTE SENSING OF ENVIRONMENT, 202, 28–44.
2017 article
Parallel Processing over Spatial-Temporal Datasets from Geo, Bio, Climate and Social Science Communities: A Research Roadmap
2017 IEEE 6TH INTERNATIONAL CONGRESS ON BIG DATA (BIGDATA CONGRESS 2017), pp. 232–250.
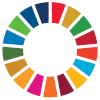
2017 journal article
Semantics-Enabled Framework for Spatial Image Information Mining of Linked Earth Observation Data
IEEE JOURNAL OF SELECTED TOPICS IN APPLIED EARTH OBSERVATIONS AND REMOTE SENSING, 10(1), 29–44.
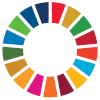
2017 conference paper
Semi-supervised deep generative models for change detection in very high resolution imagery
2017 ieee international geoscience and remote sensing symposium (igarss), 1063–1066.
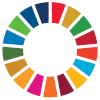
2016 article
A Scalable Probabilistic Change Detection Algorithm for Very High Resolution (VHR) Satellite Imagery
2016 IEEE INTERNATIONAL CONGRESS ON BIG DATA - BIGDATA CONGRESS 2016, pp. 275–282.
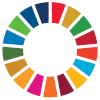
2016 journal article
Detecting Extreme Events in Gridded Climate Data
Procedia Computer Science, 80, 2397–2401.
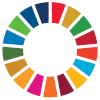
2016 article
Guest editorial: big spatial data
Vatsavai, R., & Chandola, V. (2016, October). GEOINFORMATICA, Vol. 20, pp. 797–799.
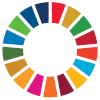
2016 journal article
Mapping Magnetic Ordering With Aberrated Electron Probes in STEM
Microscopy and Microanalysis, 22(S3), 1676–1677.
2016 journal article
Monitoring Land-Cover Changes A machine-learning perspective
IEEE GEOSCIENCE AND REMOTE SENSING MAGAZINE, 4(2), 8–21.
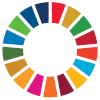
2016 conference paper
Scalable nearest neighbor based hierarchical change detection framework for crop monitoring
2016 IEEE International Conference on Big Data (Big Data), 1309–1314.
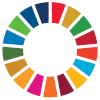
2016 journal article
Sliding Window-based Probabilistic Change Detection for Remote-sensed Images
Procedia Computer Science, 80, 2348–2352.
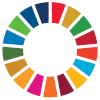
2016 article
pFUTURES: A Parallel Framework for Cellular Automaton Based Urban Growth Models
GEOGRAPHIC INFORMATION SCIENCE, (GISCIENCE 2016), Vol. 9927, pp. 163–177.
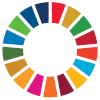
2015 article
A Scalable Complex Pattern Mining Framework for Global Settlement Mapping
2015 IEEE INTERNATIONAL CONGRESS ON BIG DATA - BIGDATA CONGRESS 2015, pp. 514–521.
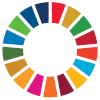
2015 conference paper
Multitemporal data mining: From biomass monitoring to nuclear proliferation detection
2015 8th International Workshop on the Analysis of Multitemporal Remote Sensing Images (Multi-Temp).
2012 journal article
Probabilistic Change Detection Framework for Analyzing Settlement Dynamics Using Very High-resolution Satellite Imagery
Procedia Computer Science, 9, 907–916.
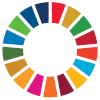
2011 journal article
A hybrid classification scheme for mining multisource geospatial data
GeoInformatica, 15(1), 29–47.
2011 journal article
A scalable gaussian process analysis algorithm for biomass monitoring
Statistical Analysis and Data Mining, 4(4), 430–445.
2011 journal article
Data Mining in Earth System Science (DMESS 2011)
Procedia Computer Science, 4, 1450–1455.
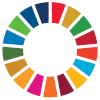
2011 journal article
GX-Means: A model-based divide and merge algorithm for geospatial image clustering
Procedia Computer Science, 4, 186–195.
2009 chapter
Incremental Clustering Algorithm for Earth Science Data Mining
In G. Allen, J. Nabrzyski, E. Seidel, G. D. van Albada, J. Dongarra, & P. M. A. Sloot (Eds.), Computational Science – ICCS 2009 (pp. 375–384).
Ed(s): G. Allen, J. Nabrzyski, E. Seidel, G. van Albada, J. Dongarra & P. Sloot
2008 chapter
A Learning Scheme for Recognizing Sub-classes from Model Trained on Aggregate Classes
In Lecture Notes in Computer Science (pp. 967–976).
2007 journal article
An efficient spatial semi-supervised learning algorithm
International Journal of Parallel, Emergent and Distributed Systems, 22(6), 427–437.
2006 chapter
Improving DB2 Performance Expert – A Generic Analysis Framework
In Lecture Notes in Computer Science (pp. 1097–1101).
2006 chapter
UMN-MapServer: A High-Performance, Interoperable, and Open Source Web Mapping and Geo-spatial Analysis System
In Geographic Information Science (pp. 400–417).
2004 chapter
Comparing Exact and Approximate Spatial Auto-regression Model Solutions for Spatial Data Analysis
In Geographic Information Science (pp. 140–161).
Employment
Updated: June 10th, 2024 15:33
2014 - present
Education
Updated: November 15th, 2019 12:49
1999 - 2008