Works (38)
2023 journal article
(Re)Use of Research Results (Is Rampant)
COMMUNICATIONS OF THE ACM, 66(2), 75–81.
Contributors: M. Baldassarre *, N. Ernst *, B. Hermann *, T. Menzies n & n
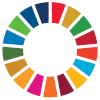
2023 journal article
An expert system for redesigning software for cloud applications
EXPERT SYSTEMS WITH APPLICATIONS, 219.
Contributors: , R. Krishna, A. Kalia, T. Menzies n , J. Xiao & M. Vukovic n
2023 journal article
How to Find Actionable Static Analysis Warnings: A Case Study With FindBugs
IEEE TRANSACTIONS ON SOFTWARE ENGINEERING, 49(4), 2856–2872.
Contributors: , H. Kang *, H. Tu *, X. Yang n, D. Lo * & T. Menzies n n
2022 article
How to Find Actionable Static Analysis Warnings: A Case Study with FindBugs
ArXiv.
2022 article
How to Improve Deep Learning for Software Analytics (a case study with code smell detection)
2022 MINING SOFTWARE REPOSITORIES CONFERENCE (MSR 2022), pp. 156–166.
Contributors: & T. Menzies n n
2022 article
How to Improve Deep Learning for Software Analytics (a case study with code smell detection)
ArXiv.
2022 article
TEXT MINING OF THE PEOPLE'S PHARMACY RADIO SHOW TRANSCRIPTS CAN IDENTIFY NOVEL DRUG REPURPOSING HYPOTHESES
MedRxiv.
Contributors: , J. Beasley *, D. Korn *, S. Abrar *, C. Melo-Filho *, E. Muratov *, J. Graedon*, T. Graedon*, R. Chirkova, A. Tropsha * *
2021 article
An Expert System for Redesigning Software for Cloud Applications
ArXiv.
2021 article
Beginning with machine learning: a comprehensive primer
Yedida, R., & Saha, S. (2021, July 21). EUROPEAN PHYSICAL JOURNAL-SPECIAL TOPICS, Vol. 7.
Contributors: & S. Saha * n
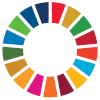
2021 report
Crowdsourcing the State of the Art(ifacts
(ArXiv Preprint No. 2108.06821).
2021 article
Crowdsourcing the state of the art(ifacts)
ArXiv.
2021 article
Documenting Evidence of a Reuse of 'A Systematic Study of the Class Imbalance Problem in Convolutional Neural Networks'
PROCEEDINGS OF THE 29TH ACM JOINT MEETING ON EUROPEAN SOFTWARE ENGINEERING CONFERENCE AND SYMPOSIUM ON THE FOUNDATIONS OF SOFTWARE ENGINEERING (ESEC/FSE '21), pp. 1595–1595.
Contributors: & T. Menzies n n
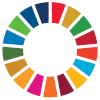
2021 article
Documenting Evidence of a Reuse of 'On the Number of Linear Regions of Deep Neural Networks'
PROCEEDINGS OF THE 29TH ACM JOINT MEETING ON EUROPEAN SOFTWARE ENGINEERING CONFERENCE AND SYMPOSIUM ON THE FOUNDATIONS OF SOFTWARE ENGINEERING (ESEC/FSE '21), pp. 1596–1596.
Contributors: & T. Menzies n n
2021 journal article
Learning to recognize actionable static code warnings (is intrinsically easy)
EMPIRICAL SOFTWARE ENGINEERING, 26(3).
Contributors: X. Yang n, J. Chen n, n , Z. Yu n & T. Menzies n
2021 conference paper
Lessons learned from hyper-parameter tuning for microservice candidate identi cation
Proceedings of the thirty-sixth IEEE/ACM International Conference on Automated Software Engineering (ASE). Presented at the 36th IEEE/ACM International Conference on Automated Software Engineering, (Virtual).
Event: 36th IEEE/ACM International Conference on Automated Software Engineering at (Virtual) on November 14-20, 2021
2021 article
Lessons learned from hyper-parameter tuning for microservice candidate identification
ArXiv.
2021 conference paper
Lessons learned from hyper-parameter tuning for microservice candidate identification
Proceedings - 2021 36th IEEE/ACM International Conference on Automated Software Engineering, ASE 2021, 1141–1145.
Contributors: , R. Krishna, A. Kalia, T. Menzies *, J. Xiao & M. Vukovic *
2021 article
Old but Gold: Reconsidering the value of feedforward learners for software analytics
ArXiv.
2021 journal article
On the Value of Oversampling for Deep Learning in Software Defect Prediction
IEEE Transactions on Software Engineering, 48(8), 1–1.
Contributors: & T. Menzies n n
2021 journal article
Simpler Hyperparameter Optimization for Software Analytics: Why, How, When
IEEE Transactions on Software Engineering, 48(8), 1–1.
Contributors: A. Agrawal*, X. Yang n, R. Agrawal n, n , X. Shen n & T. Menzies n
2021 report
When SIMPLE is better than complex: A case study on deep learning for predicting Bugzilla issue close time
(ArXiv Preprint No. 2101.06319).
2020 review
Evolution of novel activation functions in neural network training for astronomy data: habitability classification of exoplanets
[Review of ]. EUROPEAN PHYSICAL JOURNAL-SPECIAL TOPICS, 229(16), 2629–2738.
Contributors: S. Saha *, N. Nagaraj *, A. Mathur, n & S. H R
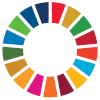
2020 article
How to Recognize Actionable Static Code Warnings (Using Linear SVMs)
ArXiv.
Contributors: X. Yang n, J. Chen n, n , Z. Yu n & T. Menzies n
2020 journal article
LipschitzLR: Using theoretically computed adaptive learning rates for fast convergence
APPLIED INTELLIGENCE, 51(3), 1460–1478.
Contributors: , S. Saha * & T. Prashanth* n
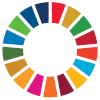
2020 article
On the value of oversampling for deep learning in software defect prediction
ArXiv.
2020 chapter
Optimizing Inter-nationality of Journals: A Classical Gradient Approach Revisited via Swarm Intelligence
In Communications in Computer and Information Science: Vol. 1290. Modeling, Machine Learning and Astronomy (Vol. 1290, pp. 3–14).
Event: Communications in Computer and Information Science at Bangalore, India on November 22-23, 2019
2020 conference paper
Parsimonious Computing: A Minority Training Regime for Effective Prediction in Large Microarray Expression Data Sets
2020 International Joint Conference on Neural Networks (IJCNN), 1–8.
Event: 2020 International Joint Conference on Neural Networks (IJCNN)
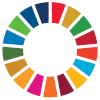
2020 article
Parsimonious computing: A minority training regime for effective prediction in large microarray expression data sets
ArXiv.
2020 report
Text Mining to Identify and Extract Novel Disease Treatments From Unstructured Datasets
(ArXiv Preprint No. 2011.07959).
2020 article
Text mining to identify and extract novel disease treatments from unstructured datasets
ArXiv.
2019 article
Evolution of novel activation functions in neural network training with applications to classification of exoplanets
ArXiv.
2019 article
LipschitzLR: Using theoretically computed adaptive learning rates for fast convergence
ArXiv.
2019 article
Simpler hyperparameter optimization for software analytics: Why, how, when?
ArXiv.
2018 speech
An Introduction to Data Analysis
Presented at the PES University, Bangalore, India.
Event: PES University at Bangalore, India
2018 article
Employee attrition prediction
ArXiv. http://www.scopus.com/inward/record.url?eid=2-s2.0-85095619264&partnerID=MN8TOARS
2018 speech
How to design a Flappy Bird game
Presented at the PES University, Bangalore, India.
Event: PES University at Bangalore, India
2018 speech
Machine Learning
Presented at the PES University, Bangalore, India.
Event: PES University at Bangalore, India
2017 speech
Complexity Classes and NP-Completeness
Presented at the PES University, Bangalore, India.