Works (3)
2022 journal article
Digital-twin-based improvements to diagnosis, prognosis, strategy assessment, and discrepancy checking in a nearly autonomous management and control system
Annals of Nuclear Energy, 166, 108715.
Contributors: L. Lin n, P. Athe n , P. Rouxelin n, M. Avramova n , A. Gupta n, *, J. Lane*, N. Dinh n
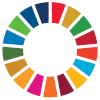
2020 journal article
Machine-learning based error prediction approach for coarse-grid Computational Fluid Dynamics (CG-CFD)
PROGRESS IN NUCLEAR ENERGY, 118.
Contributors: B. Hanna n, N. Dinh n , * & I. Bolotnov n
2019 journal article
A data-driven framework for error estimation and mesh-model optimization in system-level thermal-hydraulic simulation
NUCLEAR ENGINEERING AND DESIGN, 349, 27–45.
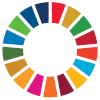