Wentao Tang
Nonlinear control, Distributed optimization, Data-driven control, Process control, Nonlinear optimization
Wentao Tang was born in Hunan Province, P. R. China. He received his B.S. degree in Chemical Engineering with a secondary degree in Mathematics and Applied Mathematics from Tsinghua University, Beijing, in 2015, and his Ph. D. degree in Chemical Engineering at University of Minnesota, Minneapolis, in 2020. He worked as a Process Control Engineer at Shell Global Solutions (U.S.) Inc., leading multiple R&D projects for the development of Shell’s advanced process control software — Platform of Advanced Control and Estimation (PACE), from 2020 to 2022. He joined the NC State University as an Assistant Professor since August 2022. He has authored over 20 journal papers and over 10 conference proceedings papers. He was a recipient of Doctoral Dissertation Fellowship of University of Minnesota for 2018—2019, and the 1st place in CAST Directors' Student Presentation Award of the 2019 AIChE Annual Meeting. His current research interests include data-driven control through machine learning, structured and scalable algorithms for distributed and black-box optimization, as well as decision making for large-scale and multi-scale systems.
Works (27)
2024 article
Data-Driven Nonlinear State Observation using Video Measurements
IFAC PAPERSONLINE, Vol. 58, pp. 787–792.
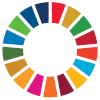
2024 article
Data-driven nonlinear state observation for controlled systems: A kernel method and its analysis
Woelk, M., & Tang, W. (2024, July 8). CANADIAN JOURNAL OF CHEMICAL ENGINEERING, Vol. 7.
2024 journal article
Systematic MPC tuning with direct response shaping: P arameterization and I nverse optimization-based T uning A pproach (PITA)
CONTROL ENGINEERING PRACTICE, 153.
2023 journal article
Automatic decomposition of large-scale industrial processes for distributed MPC on the Shell-Yokogawa Platform for Advanced Control and Estimation (PACE)
COMPUTERS & CHEMICAL ENGINEERING, 178.
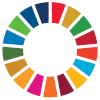
2023 article
Data-Driven State Observation for Nonlinear Systems based on Online Learning
Tang, W. (2023, May 10).
2023 journal article
Data-driven state observation for nonlinear systems based on online learning
AICHE JOURNAL, 8.
2023 article
Optimal Design of Control-Lyapunov Functions by Semi-Infinite Stochastic Programming
2023 62ND IEEE CONFERENCE ON DECISION AND CONTROL, CDC, pp. 7277–7284.
2023 article
Resolving large-scale control and optimization through network structure analysis and decomposition: A tutorial review
2023 AMERICAN CONTROL CONFERENCE, ACC, pp. 3113–3129.
2023 journal article
The future of control of process systems
COMPUTERS & CHEMICAL ENGINEERING, 178.
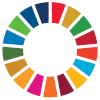
2021 journal article
Coordinating distributed MPC efficiently on a plantwide scale: The Lyapunov envelope algorithm
Computers & Chemical Engineering, 155, 107532.
2021 journal article
Fast and stable nonconvex constrained distributed optimization: the ELLADA algorithm
Optimization and Engineering, 23(1), 259–301.
2021 journal article
Nonlinear state and parameter estimation using derivative information: A Lie-Sobolev approach
Computers & Chemical Engineering, 151, 107369.
2021 journal article
Stochastic blockmodeling for learning the structure of optimization problems
AIChE Journal, 68(6).
2020 journal article
Dissipativity learning control (DLC): Theoretical foundations of input–output data-driven model-free control
Systems & Control Letters, 147, 104831.
2019 journal article
A Bilevel Programming Approach to the Convergence Analysis of Control-Lyapunov Functions
IEEE Transactions on Automatic Control, 64(10), 4174–4179.
2019 journal article
DeCODe: a community-based algorithm for generating high-quality decompositions of optimization problems
Optimization and Engineering, 20(4), 1067–1084.
2019 journal article
Decomposition of control and optimization problems by network structure: Concepts, methods, and inspirations from biology
AIChE Journal, 65(10).
2019 journal article
Dissipativity learning control (DLC): A framework of input–output data-driven control
Computers & Chemical Engineering, 130, 106576.
2019 journal article
Distributed decision making for intensified process systems
Current Opinion in Chemical Engineering, 25, 75–81.
2019 journal article
Topology Effects on Sparse Control of Complex Networks with Laplacian Dynamics
Scientific Reports, 9(1).
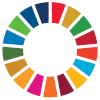
2018 journal article
Distributed adaptive dynamic programming for data-driven optimal control
Systems & Control Letters, 120, 36–43.
2018 journal article
Distributed control and optimization of process system networks: A review and perspective
Chinese Journal of Chemical Engineering, 27(7), 1461–1473.
2018 journal article
Network decomposition for distributed control through community detection in input–output bipartite graphs
Journal of Process Control, 64, 7–14.
2018 journal article
Relative time‐averaged gain array (RTAGA) for distributed control‐oriented network decomposition
AIChE Journal, 64(5), 1682–1690.
2017 journal article
Decomposing complex plants for distributed control: Perspectives from network theory
Computers & Chemical Engineering, 114, 43–51.
2017 journal article
Optimal decomposition for distributed optimization in nonlinear model predictive control through community detection
Computers & Chemical Engineering, 111, 43–54.
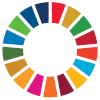
2016 journal article
Control configuration synthesis using agglomerative hierarchical clustering: A graph-theoretic approach
Journal of Process Control, 46, 43–54.
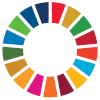
Employment
Updated: May 15th, 2022 12:17
2022 - present
2020 - 2022
2015 - 2020
2019 - 2019
Education
Updated: May 15th, 2022 12:14
2015 - 2020
2011 - 2015