Works (10)
2024 review
A Brief Review of Hemp Fiber Length Measurement Techniques
[Review of ]. FIBERS, 12(11).
2024 article
Adversarial Robustness in Graph Neural Networks: Recent Advances and New Frontier
2024 IEEE 11TH INTERNATIONAL CONFERENCE ON DATA SCIENCE AND ADVANCED ANALYTICS, DSAA 2024, pp. 433–434.
2024 conference paper
Data Quality-aware Graph Machine Learning
Wang, Y., Ding, K., Liu, X., Kang, J., Rossi, R., & Derr, T. (2024, October 21).
2024 conference paper
Linear-Time Graph Neural Networks for Scalable Recommendations
Zhang, J., Xue, R., Fan, W., Xu, X., Li, Q., Pei, J., & Liu, X. (2024, May 13).
2024 journal article
Manufacturing service capability prediction with Graph Neural Networks
JOURNAL OF MANUFACTURING SYSTEMS, 74, 291–301.
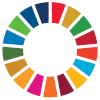
2023 article
Enhancing Graph Representations Learning with Decorrelated Propagation
PROCEEDINGS OF THE 29TH ACM SIGKDD CONFERENCE ON KNOWLEDGE DISCOVERY AND DATA MINING, KDD 2023, pp. 1466–1476.
2023 article
How does the Memorization of Neural Networks Impact Adversarial Robust Models?
PROCEEDINGS OF THE 29TH ACM SIGKDD CONFERENCE ON KNOWLEDGE DISCOVERY AND DATA MINING, KDD 2023, pp. 2801–2812.
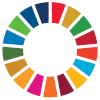
2023 article
Large-Scale Graph Neural Networks: The Past and New Frontiers
PROCEEDINGS OF THE 29TH ACM SIGKDD CONFERENCE ON KNOWLEDGE DISCOVERY AND DATA MINING, KDD 2023, pp. 5835–5836.
2022 article
Imbalanced Adversarial Training with Reweighting
2022 IEEE INTERNATIONAL CONFERENCE ON DATA MINING (ICDM), pp. 1209–1214.
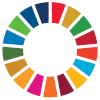
2022 journal article
Trustworthy AI: A Computational Perspective
ACM Transactions on Intelligent Systems and Technology.