2023 journal article
An Overview of and Advances in Modeling and Interoperability of Deep Neural Sleep Staging
Physiologia.
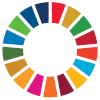
Sleep staging has a very important role in diagnosing patients with sleep disorders. In general, this task is very time-consuming for physicians to perform. Deep learning shows great potential to automate this process and remove physician bias from decision making. In this study, we aim to identify recent trends on performance improvement and the causes for these trends. Recent papers on sleep stage classification and interpretability are investigated to explore different modeling and data manipulation techniques, their efficiency, and recent advances. We identify an improvement in performance up to 12% on standard datasets over the last 5 years. The improvements in performance do not appear to be necessarily correlated to the size of the models, but instead seem to be caused by incorporating new architectural components, such as the use of transformers and contrastive learning.